Machine Learning Security Evasion Competition 2021 Calls for Researchers and Practitioners
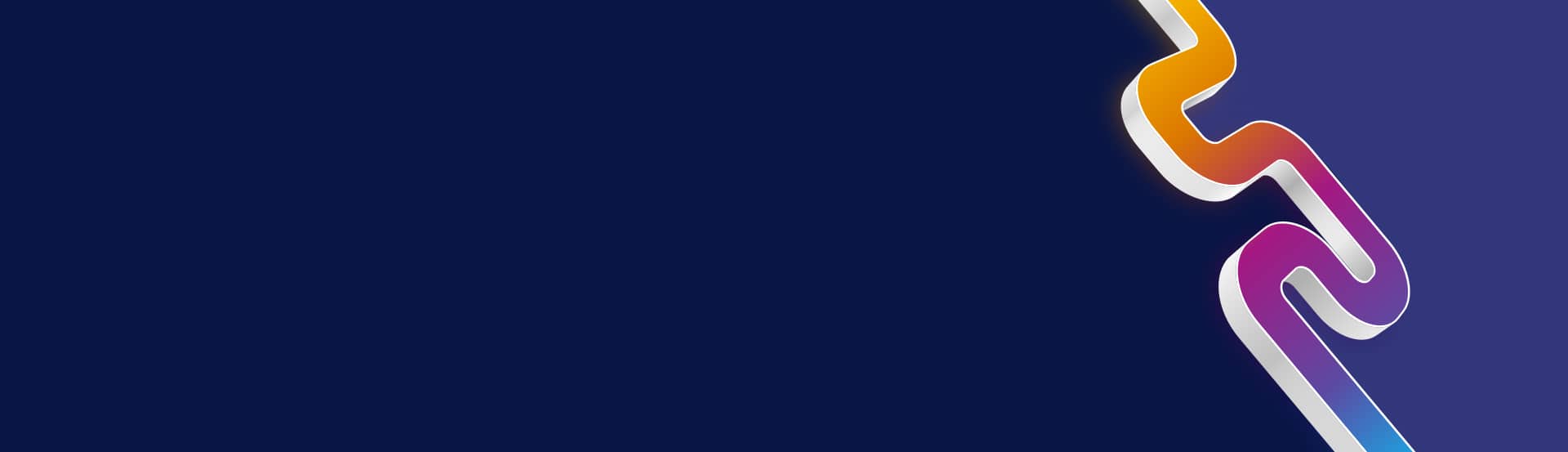
The annual machine learning (ML) security evasion competition invites ML practitioners and security researchers to compete against their peers in two separate tracks: the defender challenge and the attacker challenge.
The Machine Learning Security Evasion Competition 2021 (MLSEC2021) is a collaboration between Hyrum Anderson, Principal Architect and Ram Shankar Siva Kumar, Data Cowboy in Azure Trustworthy Machine Learning at Microsoft, Zoltan Balazs, Head of Vulnerability Research Lab at CUJO AI, Carsten Willems, CEO at VMRay, and Chris Pickard, CEO at MRG Effitas to allow researchers to exercise their defender and attacker muscles against ML security models in a unique real-world setting.
“Today, there are probably only a handful of people who are experts in both ML and cybersecurity. I am excited to be offering a unique learning opportunity for those getting started who would like to make a name for themselves in this field,” claims Balazs.
The MLSEC2021
The contest is designed to spotlight countermeasures to malicious behavior and raise awareness of the various ways in which threat actors can evade ML systems. This year, to make it easier for more people to participate, the competition will contain:
1. An anti-phishing model (from CUJO AI) in the attacker challenge.
2. Microsoft’s newly open-sourced tool Counterfit for both malware and phishing campaigns in the attacker challenge.
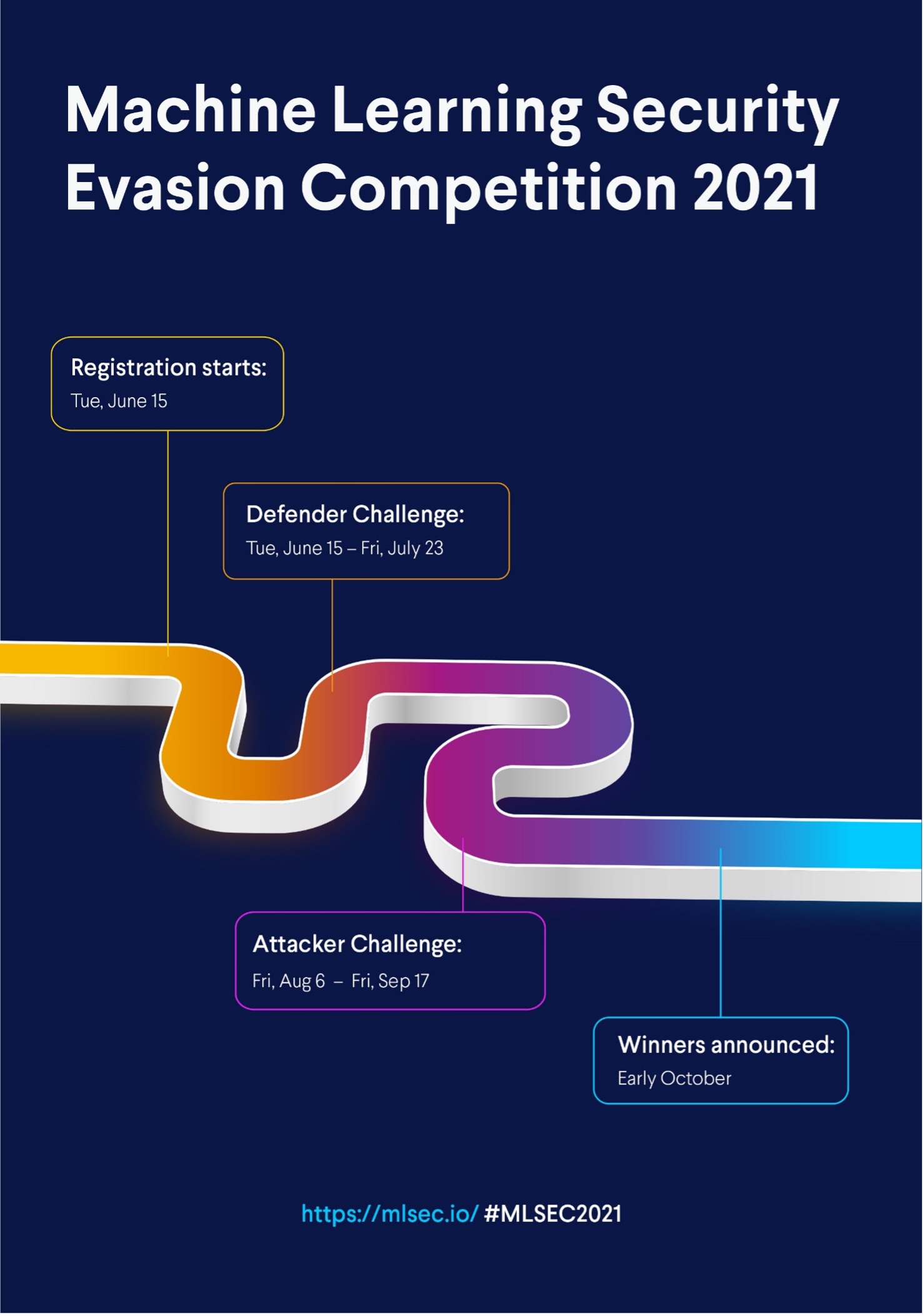
The Defender Challenge
The Defender Challenge will run from June 15 through July 23, 2021, during which contestants will submit malware detection models. These models will become targets for evasion for the subsequent Attacker Challenge. Malware models are expected to exhibit reasonable performance, and submissions will be rejected if they exceed a 1% FP rate. To win the Defender Challenge, the models must allow the fewest evasions during the subsequent Attacker Challenge round. “Last year was our first year for the Defender Challenge, and we received some incredibly encouraging solutions from contestants, particularly from academic institutions,” says Anderson. “This presents a great learning opportunity for contestants. We fully expect additional defensive measures to be employed this year that will be even more robust to adversarial manipulation of the input samples.”
The Attacker Challenge
The Attacker Challenge will run from August 6 through September 17, 2021, where researchers will modify malicious binaries in a way that preserves the binaries’ functionality, but also in a way as to not be detected by the malware detection models submitted during the Defender Challenge round. Participants will submit modified malware samples—which preserve the functionality of original samples provided to each contestant—that are intended to evade a suite of machine learning static malware detectors. Malware samples are automatically detonated in a sandbox to ensure that functionality has been preserved.
Anti-Phishing Evasion Track
New this year, the Attacker Challenge will include a Anti-Phishing Evasion track on top of the existing Anti-Malware Evasion track to allow even broader participation. An anti-phishing model provided by CUJO AI will consist of an HTML-based phishing website detector. Contestants will modify malicious phishing websites to preserve the rendering but evade the phishing ML model.
“We are introducing the phishing challenge to encourage broader participation. It is easier to modify HTML than malicious binaries, so this should allow a straightforward way for those without significant experience in malware to participate in the security evasion challenge,” says Balazs. “The phishing ML model is a custom, purpose-built ML model, created for this competition only. It was built with the goal to have multiple solutions, which are challenging enough for the competition, but easy enough to be beginner friendly.” Those wishing to conduct algorithmic attacks but who are not well-versed in adversarial ML may also leverage Microsoft’s recently announced Counterfit, an open-source tool for assessing ML model security and practice on CTF box for ML systems recently released by NVIDIA.
“As in previous years, the solution evaluation for the malware challenge will rely on the fully automated workflow of VMRay Analyzer to evaluate incoming submissions from the attacker challenge. The unique quality of the threat intel provided gives a clear view of the capabilities of the malware samples submitted by the contestants. Normally our products are used by security analysts of governments, enterprises, and the leading tech giants all around the globe, and it is a great pleasure for us to contribute to the improvement of AI-based malware detection, sponsor great academical research, and help young talent make a name for themselves in this way,” says Willems.
Register to the MLSEC2021
To participate in either challenge, individuals or teams may register at https://mlsec.io anytime during that challenge’s window. Prizes will be awarded for each challenge. To win, researchers must publish their detection or evasion strategies. The winners will be announced at the beginning of October.
Find out more about the previous Machine Learning Security Evasion Competition 2020, its results and behind the scenes.