Announcing the Winners of the 2021 MLSEC
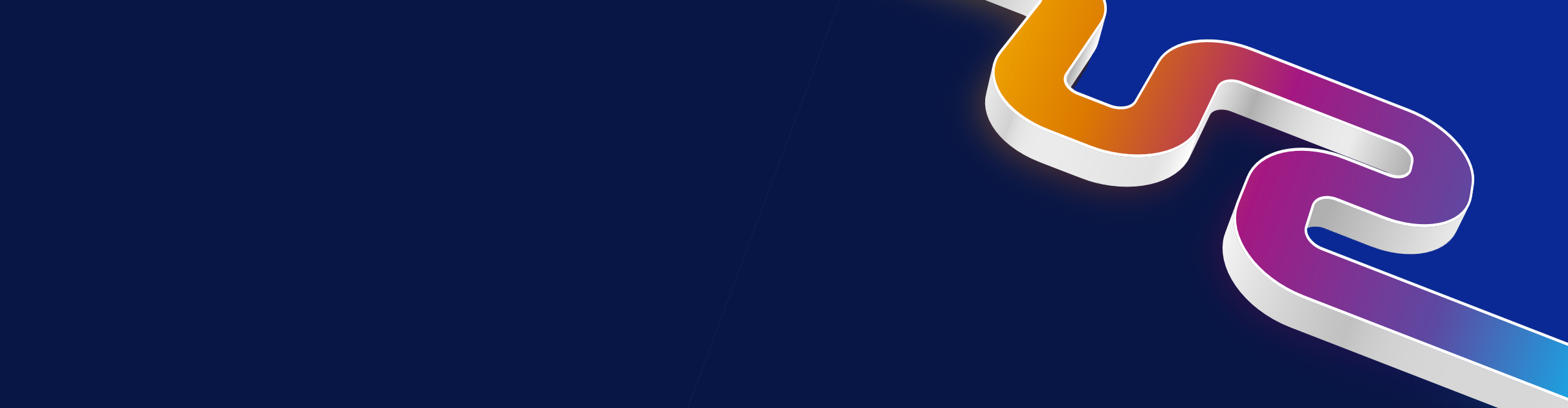
Our increasing reliance on AI systems may present an expanding attack surface to motivated adversaries. To proactively drive awareness of this issue, CUJO AI partnered with competition sponsor Microsoft and other contributors VM-Ray, MRG Effitas and NVIDIA, to host the 3rd Machine Learning Security Evasion Competition (MLSEC). The competition allowed defenders and attackers to exercise their security and machine learning skills under a plausible threat model: evading antimalware and antiphishing filters. In the competition, defenders aimed to detect evasive submissions by using machine learning (ML), and attackers attempted to circumvent those detections.
Competition Results
We are happy to announce the winners for the 2021 ML Security Evasion Competition.
Attacker Challenge: Anti-Phishing Evasion
- First place: Vladislav Tushkanov and Dmitry Evdokimov (Kaspersky)
- Second place: Ryan Reeves
Attacker Challenge: Anti-Malware Evasion
- First place: Fabrício Ceschin and Marcus Botacin (Federal University of Paraná)
- Second place: Alejandro Mosquera
- Bonus prize*: Kevin Yin
Defender Challenge (Anti-Malware)
- First place: Fabrício Ceschin and Marcus Botacin (Federal University of Paraná)
- Second place: Alejandro Mosquera
*This year, a bonus prize was awarded to any competitive attacker solution that could be automated by using the AI vulnerability assessment tool Counterfit.
Anti-Phishing Evasion. New this year, the Anti-Phishing Evasion Attacker Challenge was intended to allow participants without deep experience in the PE malware to participate. Four participants achieved a perfect evasion score of the anti-phishing models purpose-built for this competition by CUJO AI. Most contestants were able to evade the suite of antiphishing models by querying the model between 5 to 8 times, on average, for each successful evasion.
Anti-Malware Evasion. This was the first year that no one achieved a perfect score in the anti-malware evasion track. The top contestants were able to obfuscate between 25 and 32 malware samples so that they evaded all of the anti-malware models. The winning solution was also the most efficient: it required, on average, only 1 query per two model evasions (600 queries over 6 models resulting in 196 evasions). This represents a significant increase in efficiency from previous years. It is not a coincidence that the top contestants in the anti-malware evasion challenge are also the top contestants in the defender challenge – contestants used their intimate knowledge of their own defenses to gain a slight edge over the other competing teams.
Defender Challenge (Anti-Malware). This year, six anti-malware models submitted by contestants passed all the qualifications for inclusion in the context (requiring <1% false positive rate on a holdout set). And they performed admirably. Across almost 90,000 malware submissions specifically designed to evade them, the top performing models were evaded less than 0.2% of the time.
Competition Themes
The popularity of the ML Security Evasion Competition has grown. This year, more than 120 contestants registered, a 100% increase from the previous year (recall our behind-the-scenes blog post for 2020). Who participated? In a post-competition survey, over 50% of contributors reported that they participated as a team, with more than 30% of all contributions coming from a sponsored organization (e.g., corporation or university). Half identified as coming from an information security background, with the remaining participants coming from a machine learning (38%) or general software engineering (12%) background.
The anti-malware evasion challenge once again was the most popular track. Contestants’ strategies included many themes from previous years. They reported using PE obfuscation methods that included PE manipulation using LIEF, process hollowing, in-memory extraction of obfuscated payloads.
Learn More
To learn more about the security of AI systems, we recommend the following resources:
- For security analysts interested in threats against AI systems, Microsoft, in collaboration with MITRE, released an ATT&CK style AdvML Threat Matrix complete with case studies of attacks on production machine learning systems.
- For engineers and policymakers, the Berkman Klein Center at Harvard University in collaboration with Microsoft, released a taxonomy documenting various machine learning failure modes.